Shopify A/B Testing: What to Test & How to Boost Conversions

You’ve likely heard that A/B testing is a key step in improving the performance of your Shopify store. Maybe you’ve even started experimenting with some simple tests of your own, like sending the same marketing email with two different subject lines to see which performs best.
But that’s barely even scratching the surface. Read on for everything you need to know about Shopify A/B testing, including:
- Why A/B testing is so important
- How to set up A/B tests in Shopify
- Ideas and best practices for more effective A/B testing
Let’s get into it…
What is A/B Testing?
A/B testing – also known as split testing and bucket testing – is the process of testing two different versions of a “thing” to see which has the biggest positive impact on user behavior. That “thing” could be…
- A product offer
- Sales and discounts
- Menu layouts
- Email subject line copy
- CTA button color/location
- Lead capture form fields
…or pretty much any other changeable element on your Shopify store.
Done well, A/B testing helps you better understand the types of messaging and page layouts that resonate with your audience and compel them to take action – which, in turn, allows you to make data-backed improvements to your website.
Why is A/B Testing Important in E-Commerce?
A/B testing plays a crucial part in e-commerce store optimization, ensuring that any changes you make to your website are based on cold, hard audience data.
That way, you can feel confident that those changes will boost your store’s performance. It’s a whole lot better than tweaking your store’s offers, promotions, or appearance based on guesswork.
For example, Beckett Simonon is a footwear brand with a commitment to sustainability and ethical business practices. After conducting qualitative and quantitative conversion analysis, they decided to add more CSR-themed messaging across their site.
Here’s the original (AKA “control”) version of a collection page…

…and here’s the A/B test variant, which generated a 5% higher conversion rate and contributed to an annualized return on investment of 237%:

Impressive stuff, huh?
Now let’s take a deeper look at the specific benefits of Shopify A/B testing…
Increase Conversion Rates
A/B testing is a key element of conversion rate optimization.
By testing elements like CTAs, ad copy, and free shipping thresholds, you can gradually make a ton of conversion-enhancing improvements to your store.
Even a small increase in conversions can have a big impact on your bottom line – after all, a 3% uptick in conversions for a store selling $1 million of products a month = an extra $30,000 in monthly revenue 💰
Pro tip: Learn more in How To Increase Conversion Rates on Shopify.
Reduce Website Bounces
Your bounce rate is the percentage of visitors who leave your Shopify store after viewing a single web page.
A high bounce rate isn’t always a bad thing – maybe they were looking for a specific piece of information, found it, then left? And perhaps they’ll come back later to buy something? But, generally speaking, higher bounce rates are an indication that consumers aren’t engaging with your website content.
Reducing your bounce rate means more multi-page sessions. And the more pages a visitor views, the more likely they are to find a product they love.
Slash Cart Abandonments
On average, 70.19% of all online shopping carts end up being abandoned.
So reducing your cart abandonment rate can have a massive impact on your conversion rate and store revenue.
A/B testing isn’t going to reduce your abandonment rate to zero.
But it can help you understand and fix the main reasons why shoppers abandon their carts. Like, maybe your product images or descriptions don’t adequately showcase your products? Maybe your website navigation is too confusing? Or perhaps your product offers could be more compelling?
Cut Customer Acquisition Costs
Depending on your e-commerce niche, it could cost you as much as $377 to acquire a single customer.
Clearly, it’s in your best interests to bring your customer acquisition costs (CACs) down. The lower they are, the more new customers you can reach without increasing your marketing budget.
Fortunately, lower CACs are kinda like a by-product of effective A/B testing.
The actual purpose of your A/B test might be to boost your conversion rate by slashing your bounce rate or improving click-throughs from social ads (or something else entirely).
But if you achieve the desired result, you’ll also see a reduction in CACs.
Boost Average Order Value
Increasing your average order value (AOV) means you can grow your store’s revenue without having to spend a ton of money on customer acquisition.
There are lots of ways that A/B testing can help you improve AOV. For instance, try testing:
- Upsell and cross-sell offers
- Free shipping thresholds
- Product bundle discounts
Pro tip: Learn more in How to Increase Average Order Value on Shopify: 12 Proven Strategies That Work.
When To A/B Test (And When Not To)
As you can see, there are a lot of good reasons to run A/B tests.
But testing isn’t always the right solution.
Before you start planning the specifics of your first test, you need to figure out the required sample size per variation (AKA the number of store visitors who see each variant in your test).
You can work this out using a tool like ABTasty’s sample size calculator:

In the above screenshot, we’re trying to boost our (imaginary) store’s conversion rate by 25%+, with a statistical significance of 95% – which means we can be 95% confident that the result didn’t happen by chance.
To run this test effectively, ABTasty tells us we need 4,936 people to see each variant.
So if we’re running a simple A/B test with two variants, we’d need at least 9,872 people to visit our store.
Trouble is, we can’t just wait indefinitely until we hit the required sample size – we need to get there in a month or less. Because the longer you run your tests, the more likely you are to face external validity threats and sample pollution, skewing the results and leaving you open to false positives.
Which means you could end up making changes to your site based on dodgy data.
So, as a general rule, A/B testing won’t work for you if you can’t meet the sample size in 2 – 4 weeks.
Don’t worry if your traffic isn’t high enough for A/B testing – you can still gather the data you need through user testing and customer surveys.
How to Set Up and Run A/B Tests on Shopify in 7 Steps
Is your Shopify store generating enough traffic to run effective A/B tests? Congratulations – now here’s how to set them up in seven simple steps:
1. Create a Priority Order for A/B Tests
If this is your first A/B test, there are likely dozens of things you’d love to experiment with. So how do you choose where to start?
The best approach is to rank all your planned tests based on their potential impact. Because it makes sense to prioritize those that are most likely to shift the needle.
While you can’t predict the future, you can take an educated guess at the likely impact of an A/B test by using one of the following frameworks:
Impact, Confidence, Effort (ICE)
Often described as the original prioritization framework, ICE involves assigning your A/B test idea a score out of 10 for each of these factors:
- Impact: Simply put, how big of an impact do you expect this test to have on your store’s performance?
- Confidence: How certain are you that your hypothesis will be correct? (More on developing a hypothesis in the next section…)
- Effort: How easy will it be to get this test off the ground?
To create your priority order, just add up the total score for each idea and rank them from highest to lowest. Simple!
Reach, Impact, Confidence, Effort (RICE)
As you likely guessed, RICE is pretty similar to ICE, but with an extra factor thrown in:
- Reach: How many people will be affected by this test within a given period?
The definition of “reach” is a little subjective, so you’ll have to figure out what it means in the context of your A/B testing strategy. For instance, you might base your score on the average traffic figure for the page(s) in your test.
Oh, and there’s another important difference between RICE and ICE – with RICE, the scoring system works like this:
Factor | How to calculate |
Reach | Assign a number based on the test’s expected reach (e.g. this could be the total traffic you expect to generate across all pages in your A/B test) |
Impact | How big of an impact will this test have? Massive = 3X, High = 2X, Medium = 1X, Low = 0.5X, Minimal = 0.25X |
Confidence | How confident are you in your estimates? 1 = “high confidence”, 0.8 = “medium”, 0.8 = “low” |
Effort | How long will it take to get the test live? Typically measured in days |
Assign a figure for each factor, then use the following calculation to score your idea:
- (Reach × Impact × Confidence) / Effort = RICE score
Potential, Importance, Ease (PIE)
The PIE framework was developed with A/B testing in mind, although it’s also used for prioritizing other product and marketing-related tasks. Like ICE, it uses three assessment factors:
- Potential: How big of a positive impact will this test produce?
- Importance: How valuable is the traffic to the page you’re testing?
- Ease: How simple is it to implement your planned test?
Award a score out of 10 for each factor and add them together to calculate your PIE score.
2. Develop Your Hypothesis
For simplicity’s sake, we’ve spoken a lot about having “ideas” for all the different A/B tests you want to run.
But, in reality, “idea” isn’t quite the right word, because it implies an element of creativity. What you actually want is a hypothesis based on data from sources like:
- Heat maps
- Analytics tools
- Customer surveys
As well as data, you need two other things to create an A/B test hypothesis – an expectation or result and a way to measure it. Put it all together using the following framework:
👉 Because we saw [result based on qualitative or quantitative store data]...
👉 …we expect that [A/B test variant] will cause [result]...
👉 …and we’ll measure it using [metric].
For instance, imagine your store has a high abandoned cart rate. You might come up with a hypothesis like this:
“Because we saw a high volume of site searches for our shipping and return policies from customers with items in their shopping cart, we expect that summarizing our shipping and return policies in our cart drawer will reduce cart abandonments. We’ll measure it using our cart abandonment rate.”
In this example, if the variant version wins the test, our hypothesis is correct. If not, the original (“control”) version won, and we’ll stick with our original cart drawer.
3. Choose A/B Testing Software
Shopify doesn’t offer any built-in A/B testing capabilities, so you’ll have to use a third-party tool to run your own tests. Fortunately, there are plenty of options in Shopify’s App Store.
Here are the best options, based on review scores from other Shopify merchants:
Tool | Price | Review score |
OptiMonk | $0 – $249+ per month | 4.8/5 (916 reviews) |
Personizely | $19 – $149+ per month | 4.9/5 (240 reviews) |
Intelligems | $99 – $999+ per month | 4.8/5 (99 reviews) |
Elevate | $99 – $499+ per month | 4.9/5 (66 reviews) |
Visually | $15 – $80+ per month | 4.9/5 (63 reviews) |
Pro tip: For more app recommendations, check out the Best Shopify Apps to Increase Conversions.
4. Build Your A/B Tests
Having defined your hypothesis and installed your chosen A/B testing software, it’s time to set up your test.
First, you need to figure out the design of your A/B test variant. For instance, in the previous section, we planned to add details of our shipping and return policies to our cart drawer – but what, exactly, should that look like?
If you’ve got a design team, ask them to mock up some options. Or, alternatively, consider hiring a freelancer.
Then it’s simply a case of putting it all together using your A/B testing tool. The specific steps will vary based on which tool you’ve chosen – check out their support documentation if you need a helping hand.
5. Run the Test
At this point, you can click “go” on your A/B test.
But you should have some idea about how long you want it to last.
We’ve already mentioned that you should be able hit your minimum sample size in no longer than a month. However, that’s not the only consideration. You should also take into account your customers’ shopping habits: how many times do they visit your store before buying, and over what period?
Ideally, your test will span a full “buying window” (without exceeding four weeks, of course).
Say that window typically lasts a week. In that case, even if you reach your desired sample size in 24 hours, it’s worth keeping the A/B test running for a few more days.
6. Dig Into the Data
A/B testing isn’t (just) about finding a clear winner and loser from each hypothesis.
It’s also about generating enough data to help you better understand your audience.
Even if your test variant performed worse than the control, we bet it resonated with certain buyers. So dive into your analytics and check the results for different segments, such as:
- New vs returning visitors
- First-time vs repeat customers
- Android vs Safari visitors
- Desktop vs mobile users
- Logged-in vs logged-out visitors
That way, you can figure out which messaging and promotions resonate with different types of customers, which can help guide your future campaigns and promotions.
7. Archive the Test
Chances are, A/B testing isn’t your sole focus.
With so much else going on, it’s easy to lose track of which tests you’ve run (and what happened). If you don’t keep track of your previous hypotheses and results, it’s easy to end up running the same tests over and over again – and that’s just a huge waste of time.
Most A/B testing tools will keep a record of the tests you’ve run. But then you’ll have to keep paying for the tool to retain access to your results.
For that reason, it’s also worth creating a simple spreadsheet to track:
- Each hypothesis you tested
- Screenshots of/links to the control and variant versions for each test
- The results of each test (i.e. which variant won?)
- Any further insights you learned from analyzing the test data
3 Ideas for Impactful Shopify A/B Tests
Even if you have a tiny website with a handful of products, there are likely countless things you can A/B test, covering everything from your store’s appearance and navigation to the discounts and offers you run (and how you promote them). Here are some potential areas to focus on…
Product Badges
Product badges are a great way to promote product offers and highlight desirable features to help your products sell faster.
Combine product badges with A/B tests to maximize your results. Here are some product badge A/B tests you can leverage in your store today:
- Show different product offers, such as "10% OFF" or "Free Shipping" badges
- Highlight desirable features like "Exclusive" or "Limited Edition" badges
- Use social proof to boost conversions with "Best Seller" or "Trending Now" badges
- Promote scarcity to capture more sales with "Almost Gone" or "Low Stock" badges
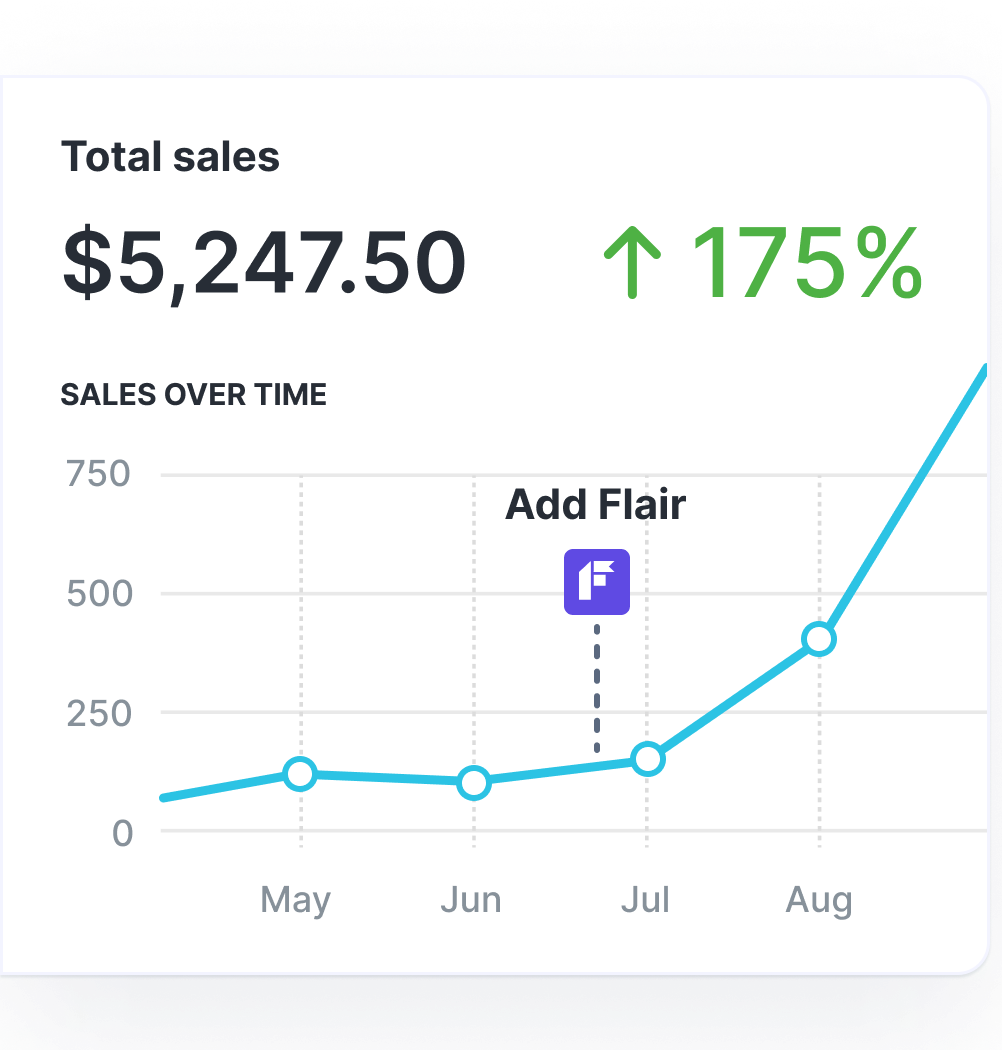
Grow Your Shopify Sales by over 175% with Flair
-
Increase sales using product badges and sales banners
-
Maximize conversions with scarcity, urgency and countdown timers
-
Automate promotions with targeted rules and scheduling
Promotions
A/B testing isn’t just about how your store looks – it’s also about the promotions you run (and how you position them).
For instance, you can experiment with the items you include in discounted product bundles, or track how different free shipping thresholds affect your overall revenue.
Pro tip: Learn more in Ignite Sales with 14 Dynamic Online Promotion Tactics!
Email Subject Lines
Subject lines are an absolute staple of A/B testing because they’re super simple to test. And, because email marketing generates a return of $36 for every $1 spent, experimenting with your email subject lines can have a big impact on your results. Try testing:
- Personalization: Does mentioning the customer’s name in the subject line increase open rates?
- Emoji usage: Are customers more likely to click when the subject line contains an emoji?
- Length: What’s the sweet spot for subject line character count?
Best Practices for Stronger A/B Testing
You might be gearing up to run your first A/B test – but you’re walking in the footsteps of millions of other Shopify merchants. That means you can learn from their successes (and failures) by following tried-and-trusted A/B testing best practices, such as…
Test One Variable At a Time
If you only take one lesson from this article, let it be this one.
You might think you’re being super efficient by tweaking the location, style, and text of your CTA button variant. But, in reality, all you’re doing is clouding the results. If your variant ends up winning, you don’t know which of your changes caused the effect – so you’re in no better position than before you ran the test 🤦♂️
By contrast, when you test a single variable at a time, you know exactly what’s going on.
And if you absolutely must test several variables at once, don’t run an A/B test – choose multivariate testing. Just bear in mind that not all A/B software supports multivariate campaigns.
Use a Large Enough Sample Size
Sure, we’ve already mentioned sample size. But it’s such a big issue that we’re repeating it here.
Simply put, if you don’t meet the required sample size for your A/B test, you can’t trust the results.
Your hypothesis could end up winning by random chance – and do you really want to make major changes to your Shopify store based on nothing more than blind luck?
Time Your A/B Tests Effectively
Again, we’ve discussed the importance of timing, but it’s absolutely essential to the effectiveness of your A/B tests – so we’re mentioning it again here.
There are a couple different time-related best practices to consider:
- Sample size: Run your test for long enough to hit your minimum sample size – but don’t keep it running longer than four weeks.
- Buying windows: Try to replicate the typical length of time it takes a customer to buy, from their first store visit to the point when they convert.
- External factors: Keep these to a minimum by running your A/B test during a “normal” period, avoiding high-traffic events like Black Friday/Cyber Monday.
Consider User Segments
Be sure to look at audience segments when analyzing the results of your A/B tests – because what appeals to returning customers may not play so well with first-timers (and so on).
That way, even if your hypothesis ends up being proven wrong, you can still see whether it resonates with specific types of customers. This can help improve your messaging and targeting for future promotional campaigns.
Focus on High-Impact A/B Tests
You might have heard that Google once tested 41 shades of blue for its search result links to see which generated the highest click-through rate.
But while this sort of granular focus can be worthwhile for a website with hundreds of millions of daily visitors, it’s likely not the best way to run A/B tests for your Shopify store.
Rather than testing minor design changes, focus on things that affect your most impactful metrics – think conversion rate, average order value, and store revenue.
Repeat A/B Tests Over Time
Sure, you don’t want to test the same hypotheses over and over again.
But your brand and audience will change over time – and what works today might not achieve the same results tomorrow.
For that reason, it’s worth repeating your highest-impact tests every year or so to make sure you’re basing optimization decisions on current data.
Factor Context Into Test Results
Remember: A/B tests don’t happen in a vacuum.
Your hypothesis might be designed to improve a specific metric, but it might also have a knock-on effect on other metrics. Make sure you take a holistic view of performance when you’re analyzing test results.
For instance, let’s say you’ve doubled the length of a product description with the goal of reducing bounce rate across all product pages – more content = more engaging, right?
Just as you suspected, your new, content-heavy variant wins the test by achieving a lower bounce rate… But it also sees a lower conversion rate.
In that case, you might have solved one problem while creating another.
You’ll have to take a closer look at the numbers to see which variant generated the highest revenue.
(Plus you’ll want to look at how these results vary across different audience segments.)
FAQs
What is the purpose of A/B testing?
The purpose of A/B testing is to test how changes to a website’s layout, features, or content can affect performance. This helps website owners to learn what types of optimizations have the biggest impact on user behavior.
What’s an example of A/B testing?
An example of A/B testing is trialing different versions of a product page:
- Version #1: The original, known as the “control” version.
- Version #2: A variant with one change compared to the control (like larger product images or a more prominent CTA button).
The test would have a predefined hypothesis – such as predicting that adding larger product images will boost conversion rates. If the hypothesis wins the test, you could then roll out the expanded product images across your other product pages.
Does Shopify allow A/B testing?
Yes, Shopify does allow A/B testing, but you’ll need to leverage one of the third-party A/B testing tools in the Shopify App Store. Examples include: